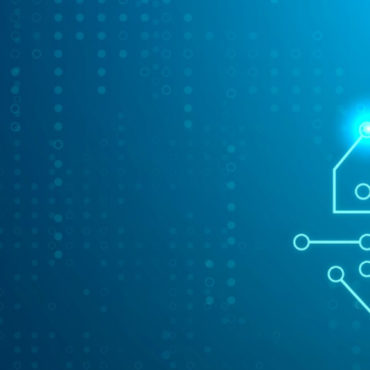
Secure AI in the Military: How Mistakes Turn Deadly
The first country to fully harness artificial intelligence (AI) for military applications will be the one that leads the world in AI warfare – according to Jack Shanahan, at least. ...
Secure AI Weekly admin todayAugust 23, 2022 187
TechTalks, August 15, 2022
Machine learning is becoming part of most of applications we use every day with increasing attention being paid to identifying and mitigating threats to the security and privacy of machine learning models. Yet some areas of machine learning security remain under-researched. One of such unexplored area is that of the security of reinforcement learning algorithms.
Researchers at McGill University and the University of Waterloo focus specifically on privacy threats of deep reinforcement learning algorithms. According to the results of the study, attackers can carry out effective attacks on deep learning systems and potentially obtain sensitive information used in training models. These results are important as reinforcement learning is implemented in industrial and consumer applications.
Adversarial examples, a more known type of attacks against machine learning, concentrate on modifying the behavior of machine learning models and pose a security risk. Membership inference attacks concentrate on extracting information from models and bring a privacy risk, as these are methods, which try to get machine learning models to report data about their training set.
InfoQ, August 15, 2022
According to a recent NCC Group’s white paper, there are internal security concerns for organizations building and deploying machine learning solutions. This document provides a classification with examples of attacks based on widely used libraries such as the SciKit-Learn, Keras, PyTorch, and TensorFlow platforms.
According to NCC Group, machine learning systems are subject to certain forms of attack that may attempt to use infrastructure or application bugs, or other existing problems. For example, many machine learning models comprise code that is performed when the model is loaded or when a certain condition is met, such as predicting a given output class. And an attacker can create a model with malicious code for various purposes, including leaking sensitive information, installing malware, generating output errors, etc. Another example is an adversarial perturbation attack, where an attacker can create an input that causes the machine learning system to return results of its choice. And many other types of attacks: membership inference attacks, model inversion attacks, data poisoning backdoor attacks.
The white paper provides a comprehensive classification of machine learning attacks, including possible mitigation measures, as well as an overview of more traditional security issues that have been detected in a lot of machine learning systems.
VentureBeat, August 16, 2022
Credit reporting agency Equifax faced a problem with its systems between March 17 and April 6, 2022 that resulted in incorrect consumer credit ratings being reported. Equifax described the issue as a “coding issue,” but there was an assumption that the issue was related to the company’s artificial intelligence systems that helped calculate credit scores.
Thomas Robinson, VP of Strategic Partnerships and Corporate Development at Domino Data Lab, said: “When it comes to Equifax, there is no shortage of finger-pointing. But from an artificial intelligence perspective, what went wrong appears to be a classic issue, errors were made in the data feeding the machine learning model.” He also added that errors could have occurred, among other things, due to incorrect label updates, incorrect manual data entry from the source, or due to inaccuracy of the data source.
Krishna Gade, co-founder and CEO of Fiddler AI, pointed out that there was another possibility , which is data drift. It can be caused by changes in the world, modifications in product usage, or data integrity issues such as bugs and degraded application performance. According to Gade, data drift incidents occur because the machine learning process assumes that the future will be the same as the past.
“Playtime is over for data science. More specifically, for organizations that create products with models that are making decisions impacting people’s financial lives, health outcomes and privacy, it is now irresponsible for those models not to be paired with appropriate monitoring and controls.” – Robinson said.
Stay up to date with what is happening! Get a first look at news, noteworthy research and worst attacks on AI delivered right in your inbox.
Written by: admin
Articles admin
The first country to fully harness artificial intelligence (AI) for military applications will be the one that leads the world in AI warfare – according to Jack Shanahan, at least. ...
Adversa AI, Trustworthy AI Research & Advisory