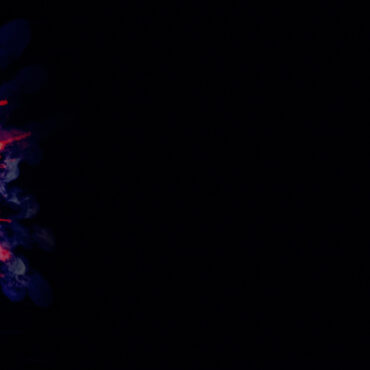
Towards Trusted AI Week 37 – Why AI TRiSM is Essential
Tackling Trust, Risk and Security in AI Models Gartner, September 5, 2023 The surge of interest in generative AI technologies has led to a plethora of pilot projects, but what ...
Trusted AI Blog + Adversarial ML admin todaySeptember 18, 2023 182
With technology advances the ethical, security, and operational questions loom ever larger. From hijacked images that can control AI to camouflage techniques that can make vehicles invisible to sensors, the latest batch of research papers unveils some startling vulnerabilities in AI systems.
Can anyone hack an AI model by just tweaking an image? Could a vehicle vanish from a self-driving car’s “sight”? Hold onto your firewalls as we dive into four groundbreaking studies that pose intriguing questions and challenge the security paradigms of various AI technologies!
The objective of this research is to assess the security vulnerabilities in foundation models, particularly vision-language models (VLMs), by exploring the concept of image hijacks — adversarial images that can control generative models at runtime.
The researchers introduce a general method called Behavior Matching to create these image hijacks and use it to test three types of attacks: specific string attacks, leak context attacks, and jailbreak attacks. These attacks were carried out against LLaVA-2, a state-of-the-art VLM, achieving a success rate of over 90% for all attack types. The study also underscores the risks these vulnerabilities pose, such as spreading malware, stealing sensitive information, and jailbreaking model safeguards, emphasizing the urgent need for security research in multimodal models.
The paper contributes to the field by exposing critical security vulnerabilities in foundation models, thereby emphasizing the need for proactive measures to understand and mitigate these risks.
This research investigates the limits of adversarial attacks on image classifiers, specifically focusing on the concept of multi-attacks, where a single adversarial perturbation can affect the classification of multiple images.
Researchers designed a single adversarial perturbation, called P, that could change the classes of hundreds of images at once, altering each to an arbitrarily chosen target class. They found that such multi-attacks are easy to generate, especially as image resolution increases, and that classifiers trained on random labels are more susceptible to these attacks. Ensembling multiple models was shown to decrease this susceptibility.
The contribution of this research lies in highlighting the scale of redundancy and vulnerability in neural network classifiers, thereby emphasizing the need for further investigation and more robust defensive strategies against adversarial attacks.
The study aims to overcome the limitations of existing adversarial camouflage methods in terms of universality, transferability, and performance with the introduction of a new framework called Adversarial Camouflage for Transferable and Intensive Vehicle Evasion (ACTIVE).
The ACTIVE framework employs a variety of innovative techniques such as Triplanar Mapping for robust and universal texture application, a novel Stealth Loss function to render vehicles undetectable, and additional loss functions to enhance the naturalness of the camouflage. Extensive experiments conducted on 15 different models demonstrated that ACTIVE significantly outperforms existing solutions across various benchmarks, including the latest YOLOv7, and shows promising transferability across different vehicle types, tasks, and real-world applications.
The key contribution of this research is the development of an advanced, universal, and transferable adversarial camouflage framework that performs exceptionally well under various conditions and scenarios.
This research provides a comprehensive overview of the security threats and vulnerabilities associated with large language models (LLMs), focusing on potential misuse such as fraud, impersonation, and malware generation. Researchers created a taxonomy that outlines the relationship between the generative capabilities of LLMs, the preventive measures designed to mitigate threats, and the vulnerabilities that arise from imperfect preventive strategies. They found that LLMs can be significantly misused in both academic and real-world settings, and emphasized the necessity of peer review in identifying and prioritizing relevant security concerns.
The contribution of this paper is its comprehensive mapping of the threat landscape associated with LLMs, serving as a foundational resource to increase awareness and inform both developers and users of these technologies about security risks.
As a wrap up, one resounding theme is clear. Vulnerabilities exist at every corner, whether in image classifiers, generative models, physical objects, or language models. While each paper uniquely contributes to its specific sub-field — be it the vulnerabilities in vision-language models, large-scale multi-attacks, 3D physical camouflage, or the ethical implications of large language models — they all underline one common message: there is an urgent need for robust security measures.
So, what sets these papers apart? It’s the methods of attack and the potential impacts from subtle image tweaks to full-scale 3D camouflage, the scale and implications vary, but the message remains the same — our AI isn’t as invincible as we thought.
Written by: admin
Secure AI Weekly admin
Tackling Trust, Risk and Security in AI Models Gartner, September 5, 2023 The surge of interest in generative AI technologies has led to a plethora of pilot projects, but what ...
Adversa AI, Trustworthy AI Research & Advisory