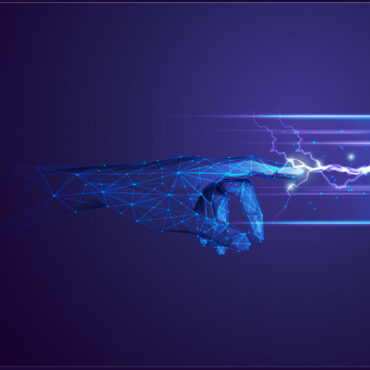
Towards Trusted AI Week 6 – The Future of AI Security
Navigating the Growth of Ethical AI Solutions in the new EAIDB Report FY 2022 EAIDB, January 31, 2023 The news media on a daily basis is awash with reports about ...
Adversarial ML admin todayFebruary 8, 2023 79 5
This monthly digest highlights the latest research papers on the security of AI. In this edition, we explore four groundbreaking papers that shed light on the vulnerabilities and adversarial attacks in AI systems. Read on to learn more about these exciting developments.
In this paper, the researchers Debayan Deb, Vishesh Mistry, and Rahul Parthe discuss the vulnerability of face recognition systems to adversarial attacks, specifically small imperceptible changes to face samples. While deep learning models have achieved high recognition rates, the presence of adversarial perturbations can evade these systems. The work highlights the potential threat of adversarial attacks in various scenarios, including fingerprint recognition systems.
The authors propose an automated adversarial synthesis method, called AdvBiom, which generates visually realistic adversarial face images that can evade state-of-the-art automated biometric systems. The method is shown to be model-agnostic, transferable, and successful against current defense mechanisms. Furthermore, the authors demonstrate how AdvBiom can be extended to evade automated fingerprint recognition systems by incorporating Minutiae Displacement and Distortion modules.
In the research paper, the authors investigate the vulnerability of code models to backdoor attacks. They propose a method called AFRAIDOOR (Adversarial Feature as Adaptive Backdoor) that leverages adversarial perturbations to inject adaptive triggers into model inputs. The study evaluates AFRAIDOOR on three widely used code models (CodeBERT, PLBART, and CodeT5) and two tasks: code summarization and method name prediction.
The results reveal that existing backdoor attacks on code models use unstealthy and easy-to-detect triggers. However, AFRAIDOOR achieves stealthiness by bypassing the detection in the defense process, with approximately 85% of adaptive triggers successfully evading detection. In contrast, less than 12% of the triggers from previous work bypass the defense. The success rates of baseline methods significantly decrease to 10.47% and 12.06% when a defense is applied, while AFRAIDOOR maintains success rates of 77.05% and 92.98% on the code summarization and method name prediction tasks, respectively.
These findings expose security weaknesses in code models under stealthy backdoor attacks and demonstrate that the current state-of-the-art defense methods are insufficient.
This study focuses on the vulnerability of wild images to backdoor poisoning, which refers to the injection of malicious code or trojans into machine learning models trained on these images.
While previous attacks assumed that the wild images are labeled, in reality, most images on the web are unlabeled. The research investigates the impact of backdoor images without labels in the context of semi-supervised learning (SSL) using deep neural networks. The adversary is assumed to have zero-knowledge, and the SSL model is trained from scratch to ensure realism.
It is observed that backdoor poisoning fails when unlabeled poisoned images come from different classes compared to poisoning labeled images. This failure occurs because SSL algorithms tend to correct such poisoned images during training. As a result, for unlabeled images, backdoor poisoning is implemented using images from the target class. To achieve this, a gradient matching strategy is proposed to craft poisoned images whose gradients match the gradients of target images in the SSL model. This strategy enables fitting the poisoned images to the target class and injecting the backdoor.
To the best of the authors’ knowledge, this may be the first approach to backdoor poisoning on unlabeled images in trained-from-scratch SSL models. Experimental results demonstrate that this poisoning technique achieves high attack success rates on most SSL algorithms while bypassing modern backdoor defenses.
The final paper explores semantic adversarial attacks on face recognition systems.
The proposed work introduces a new Semantic Adversarial Attack using StarGAN called SAA-StarGAN that aims to manipulate significant facial attributes, generating effective and transferable adversarial examples. Previous works in this area have focused on changing single attributes without considering the intrinsic attributes of the images. SAA-StarGAN addresses this limitation by identifying and tampering with the most significant facial attributes for each image.
In the white-box setting, the method predicts the most important attributes using either cosine similarity or probability scores based on a Face Verification model. These attributes are then modified using the StarGAN model in the feature space. The Attention Feature Fusion method is used to generate realistic images, and different values are interpolated to produce diverse adversarial examples. In the black-box setting, the method relies on predicting the most important attributes through cosine similarity and iteratively altering them on the input image to generate adversarial face images.
Experimental results show that predicting the most significant attributes is crucial for successful attacks. SAA-StarGAN achieves high attack success rates against black-box models, outperforming existing methods by 35.5% in impersonation attacks. The method also maintains high attack success rates in the white-box setting while generating perceptually realistic images that avoid confusing human perception. The study confirms that manipulating the most important attributes significantly affects the success of adversarial attacks and enhances the transferability of the crafted adversarial examples.
The contributions of this work include the proposal of SAA-StarGAN, which enhances the transferability of adversarial face images by tampering with critical facial attributes. The method effectively generates semantic adversarial face images in both white-box and black-box settings, using different techniques for impersonation and dodging attacks. Modifications are proposed to adapt SAA-StarGAN for the black-box setting, and empirical results demonstrate its superior performance in terms of attack success rate and adversarial transferability while maintaining perceptually realistic images.
These research papers shed light on the vulnerabilities of AI systems, including biometric matchers, code models, semi-supervised learning models, and face recognition systems. The findings emphasize the need for robust defense mechanisms to mitigate the risks posed by adversarial attacks.
Written by: admin
Secure AI Weekly admin
Navigating the Growth of Ethical AI Solutions in the new EAIDB Report FY 2022 EAIDB, January 31, 2023 The news media on a daily basis is awash with reports about ...
Adversa AI, Trustworthy AI Research & Advisory