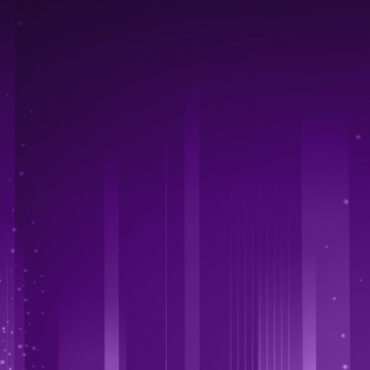
Prompt Engineering and LLM Security Digest for May 2023
With this monthly digest, feel free to discover the power of ChatGPT! Learn how to explore the best ChatGPT plugins, dive into Generative AI, and master prompt engineering. Click on ...
Trusted AI Blog + Adversarial ML admin todayJune 5, 2023 28
Dive into the intricate tapestry of newest artificial intelligence research as we unravel a series of compelling Arxiv papers spanning diverse topics ranging from neurosymbolic AI, autonomous drone manipulation to real-world vulnerabilities in language model applications.
The essence of each study lies within the careful blend of objectives, methodologies, findings, and contributions, painting a broader picture of the ever-evolving AI landscape.
The research paper authored by Amit Sheth, Kaushik Roy, and Manas Gaur focuses on Neuro-Symbolic Artificial Intelligence (AI).
The objective of the research is to explore the capabilities and implications of Neurosymbolic AI, a paradigm that combines neural networks with symbolic knowledge structures, in enhancing both the algorithmic and application-level functions of AI systems. Neuro-Symbolic AI is not new, but interest in it has surged recently due to major advancements in machine learning, particularly through deep learning, which has led to a considerable increase in research and practical applications.
The researchers conducted comparative studies on different neurosymbolic architectures, focusing on their capabilities in perception and cognition, scalability, and support for user-explainability and continual learning.
The results revealed that combining language models with knowledge graphs not only offers current utility but also has future potential for modeling diverse types of application and domain-level knowledge.
The significant contribution of this research lies in its detailed assessment and optimistic outlook for Neurosymbolic AI, emphasizing its potential for enhanced explainability, safety, and regulatory compliance in various application areas.
The research aims to evaluate the robustness of large vision-language models (VLMs) like GPT-4, particularly in a realistic and high-risk setting, where adversaries can subtly manipulate the most vulnerable modality (such as vision) to evade the system.
What Researchers Did Exactly: The researchers crafted targeted adversarial examples against pretrained models like CLIP and BLIP, and transferred these examples to other VLMs, while also studying how black-box queries on these models could further improve the effectiveness of targeted evasion.
Results Found: The study found a surprisingly high success rate in generating targeted responses through the use of adversarial examples, revealing a quantitative understanding of the adversarial vulnerability of large VLMs and the dependency of system robustness on their most vulnerable input modality.
The research offers a comprehensive examination of potential security flaws in large VLMs, contributing valuable insights and advocating for thorough security assessments prior to deploying these models, thus calling attention to a critical aspect of modern multimodal systems.
The research aimed to develop and analyze flying adversarial patches that can be mounted on a multirotor (attacker UAV) to manipulate the neural network’s predictions of another multirotor (victim UAV), thereby gaining control over its motions.
The researchers introduced flying adversarial patches and compared three methods for simultaneously optimizing the adversarial patch and its position in the victim’s input image, conducting empirical validation on a publicly available deep learning model and dataset for autonomous multirotors.
Their empirical studies revealed that the hybrid optimization approach outperformed other methods, and the calculated adversarial patch could successfully shift the neural network’s predictions to desired values, with the best results achieved for up to 4 desired target positions.
This research represents an innovative advancement in adversarial attack techniques, offering a novel method for manipulating and controlling Unmanned Aerial Vehicles (UAVs) through optimized adversarial patches; it highlights vulnerabilities in current deep learning models applied to critical infrastructure and suggests paths for future real-world applications and enhancements.
The research sought to explore the vulnerabilities of Large Language Models (LLMs) in various applications, particularly the susceptibility to Indirect Prompt Injection attacks, which allow adversaries to remotely exploit the applications by injecting strategic prompts into retrieved data.
The authors introduced the concept of Indirect Prompt Injection (IPI) to compromise LLM-integrated applications, identified new attack vectors, developed a comprehensive taxonomy from a computer security perspective to systematically examine potential impacts and vulnerabilities, and demonstrated the practical feasibility of these attacks on both real-world systems like Bing’s GPT-4 and synthetic applications built on GPT-4.
Their findings showed that processing retrieved prompts can act like arbitrary code execution, manipulate the application’s functionality, and control API calls, potentially leading to full compromise of the model at inference time, remote control, persistent compromise, data theft, and denial of service, among other serious threats.
This research provides the first investigation into the threat landscape associated with IPI in LLM-integrated applications, contributing a novel concept and taxonomy, along with practical demonstrations and insights into the implications of these threats, thereby establishing a foundation for the urgently-needed security evaluation of LLM-integrated applications and stimulating the development of robust defenses.
The researchers shared all demonstrations on their GitHub repository and developed attack prompts in the Appendix of this paper to foster future research.
These research papers provide a kaleidoscopic view of the fascinating yet complex world of AI research, unveiling the immense potential and the intricacies that lie within. Despite their diverse focus areas – from neurosymbolic AI to adversarial resilience, drone behavior manipulation, and LLM vulnerabilities, a common thread that weaves them together is the ubiquitous need for robustness and security in AI applications.
Each paper thus opens a new avenue for further exploration, inching us closer to a future where AI is not just advanced, but also secure, interpretable, and reliable.
Written by: admin
Trusted AI Blog admin
With this monthly digest, feel free to discover the power of ChatGPT! Learn how to explore the best ChatGPT plugins, dive into Generative AI, and master prompt engineering. Click on ...
Adversa AI, Trustworthy AI Research & Advisory