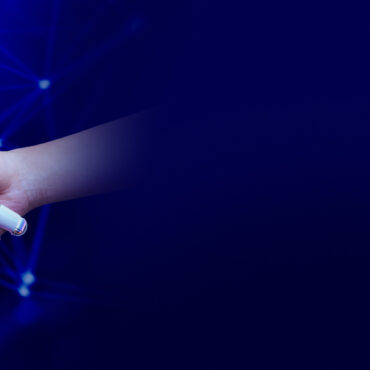
ChatGPT digest: From AI Ethics to Cheating
A Student Used ChatGPT to Cheat in an AI Ethics Class Gizmodo, February 18, 2023 Using artificial intelligence to cheat in academic settings is not a new phenomenon. However, recent ...
Adversarial ML admin todayMarch 2, 2023 66
This digest describes four research papers that investigate AI security, specifically focusing on ways AI systems can be tricked or compromised, and how to make them more resistant to these attacks.
This research aims to investigate the adversarial robustness of federated learning systems. The researchers meticulously examined various federated learning algorithms and their responses to different adversarial attacks.
The study revealed that federated learning systems, while resilient in certain conditions, are vulnerable to tailored adversarial attacks. It provides invaluable insights into the limitations of federated learning systems and lays the foundation for the development of more secure and robust models.
The paper seeks to explore a novel approach to adversarial attacks by incorporating raindrops as perturbations. Researchers developed a new technique to simulate raindrops and used them as a physical-world disguise to launch adversarial attacks on image classification systems.
The paper demonstrated that simulated raindrops can be effectively used to fool state-of-the-art image classifiers. This work highlights a real-world example of adversarial attacks and contributes to a better understanding of physical-world vulnerabilities in machine learning systems.
The objective of this paper is to exploit scientific plots as a novel means of executing information-stealing attacks on machine learning models.
Researchers devised an innovative approach that extracts valuable information from machine learning models by analyzing their scientific plots. The study successfully demonstrated the extraction of sensitive information from machine learning models through scientific plots.
The paper introduces an unconventional method of model information extraction and serves as a reminder of the importance of safeguarding scientific plots against malicious actors.
This research focuses on evaluating ChatGPT’s robustness against adversarial inputs and out-of-distribution samples. Researchers conducted extensive testing of ChatGPT by subjecting it to a battery of adversarial and out-of-distribution samples. ChatGPT demonstrated notable robustness but exhibited vulnerabilities when confronted with certain adversarial inputs.
This study is among the pioneers in evaluating ChatGPT’s adversarial robustness and provides important insights for its further improvement and safe deployment.
As we conclude this journey through the realm of adversarial ingenuity, it becomes apparent that all these papers converge on exploring vulnerabilities and robustness in machine learning systems. Whether it’s through novel adversarial attacks with raindrops, analyzing scientific plots, or evaluating cutting-edge federated learning systems and language models like ChatGPT, they all shine a light on the darker side of artificial intelligence.
However, the approaches they employ vary from real-world physical simulations to meticulous algorithmic analyses. These differences remind us that while AI systems possess immense potential, they are not infallible, and their security is an ongoing endeavor that will shape the future of technology.
Written by: admin
Secure AI Weekly admin
A Student Used ChatGPT to Cheat in an AI Ethics Class Gizmodo, February 18, 2023 Using artificial intelligence to cheat in academic settings is not a new phenomenon. However, recent ...
Adversa AI, Trustworthy AI Research & Advisory