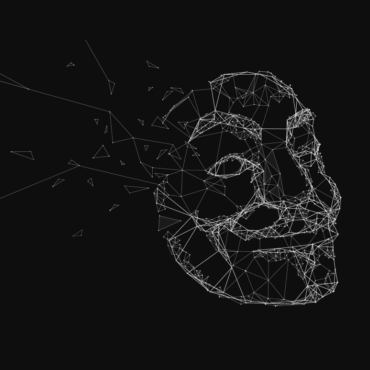
Towards trusted AI Week 48 – hackers’ benefits from AI
Ensuring AI security is not an easy thing, and a lot is to be done in the future. Making attacks easier with the help of AI TechHQ, November 23, 2020 ...
Adversarial ML admin todayNovember 30, 2020 105
This month, as usual, we have prepared for you a selection of the most interesting research on the security of artificial intelligence.
Deep neural networks are used in various fields, but at the moment there is a huge amount of research confirming the vulnerability to adversarial attacks.
Earlier works mainly dealt with the adversarial attack focusing a single task, however, in reality, an attack often involves several models for different tasks. The study presented Multi-Task adversarial Attack (MTA), a unified framework capable of making adversarial examples for many tasks at a time. MTA everages shared knowledge among tasks significantly increasing the capabilities of adversarial attacks on real-world systems. The framework also has a generator for creating adversarial perturbations, including per-instance and universal ones. Research was conducted on the Office-31 and NYUv2 datasets Experts have demonstrated that MTA significantly improves the effectiveness of adversarial attacks compared to single-task setting.
To date, great results have been achieved regarding the ability of machine learning models, deep neural networks in particular, to cope with such tasks as image and speech recognition, language understanding, etc. However, such models have demonstrated their vulnerability to various attacks, one of which is poisoning. Such an attack consists in the fact that a third party injects a specially created sequence of samples to a targeted model aiming to affect its learning. In a new work, the researchers presented a completely new type of this attack called the Lethean Attack. It is a new type of poisoning, as a result of which the model experiences critical forgetting. The attack was tested under Test-Time Training, an online learning framework for generalization under distribution shifts.The researchers compared this method with other previously known sample sequences that also caused forgetting, however, in comparison with them, the new Lethean Attack has demonstrated much more efficiency.
Today, the surveillance sphere actively uses deep learning in machine vision technologies; this allows the system to produce human identification and object detection based on video, photo and audio information. Despite the fact that the technologies involved in the surveillance cameras have learned to recognize people with great accuracy, at the moment they can be successfully used for adversarial attacks that help to deceive the system in recognition.This study focuses on adsarial attacks based on the use of visible light when targeting facial recognition systems.
In the course of the work, the researchers first study the use of infrared light in an adversarial attack, then they switch to a visible light attack. The experts emphasize that the purpose of their study was to prove the vulnerability of such systems and propose ways to further protect them from adversarial attacks using light.
Written by: admin
Secure AI Weekly admin
Ensuring AI security is not an easy thing, and a lot is to be done in the future. Making attacks easier with the help of AI TechHQ, November 23, 2020 ...
Adversa AI, Trustworthy AI Research & Advisory