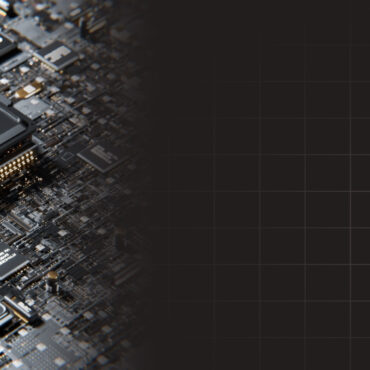
Towards Secure AI Week 14 – New AI Security Report and Hacking Grok AI
X’s Grok AI is great – if you want to know how to hot wire a car, make drugs, or worse The Register, April 2, 2024 The innovative generative AI ...
Trusted AI Blog + LLM Security admin todayApril 11, 2024 657
Welcome to our LLM Security TOP Digest!
Discover the latest news in LLM security with our selection of top articles and research findings. From innovative defense strategies to emerging threats, stay informed and learn how leading researchers and organizations are safeguarding AI systems.
Let’s start!
This IDC Innovators study highlights four emerging vendors, offering AI security solutions tailored to address the unique risks posed by AI algorithms. These vendors provide specialized protection measures to prevent, detect, and mitigate adversarial attacks on AI applications and models, complementing traditional cybersecurity technologies. Adversa AI is included in this list as one of four emerging vendors.
Over 100 malicious AI/ML models have been discovered in the Hugging Face platform, posing a significant security threat, according to the post on The Hacker News. These models, capable of executing code leading to full control over compromised machines, were identified by JFrog, raising concerns about potential large-scale data breaches or corporate espionage.
Additionally, researchers have developed attack techniques like prompt injection to manipulate large-language models (LLMs), highlighting ongoing challenges in securing AI systems and the potential for widespread misuse.
“The study is not the first, nor will it be the last, to explore the idea of prompt injection as a way to attack LLMs and trick them into performing unintended actions.”
The article discusses the importance of crafting a generative AI (GenAI) security policy to address the cybersecurity risks associated with the rapid evolution of AI technologies. It emphasizes the need for organizations to combat potential threats such as social engineering scams, prompt injection attacks, and data poisoning attacks facilitated by GenAI.
The policy outlines key considerations across people, process, technology, security operations, facilities operations, financial performance, and company performance to effectively manage and mitigate the impact of GenAI-based security breaches. This is crucial for CISOs to ensure robust cybersecurity strategies that encompass emerging AI technologies.
This blog post discusses the importance of applying relevant security controls to secure generative AI applications. It highlights the use of a Generative AI Scoping Matrix to determine the scope of the application, enabling a focused approach to implementing necessary security measures.
By mapping controls to mitigations from frameworks like MITRE ATLAS and referencing industry resources like OWASP AI Security and NIST’s AI Risk Management Framework, the article emphasizes the need for security architects, engineers, and developers to integrate secure-by-design practices into their workflows to address emerging AI-related threats and vulnerabilities effectively. This approach ensures that organizations can adapt their existing security practices to safeguard generative AI technologies without necessitating a complete overhaul of their security protocols.
The article discusses the emergence of conditional prompt injection attacks with Microsoft Copilot, highlighting the challenges and potential implications for cybersecurity. These attacks leverage the capabilities of large language models (LLMs) to execute tailored instructions based on specific user interactions.
The demonstration of different attack scenarios underscores the importance of understanding and mitigating prompt injection vulnerabilities to prevent unauthorized access, data manipulation, and potential data exfiltration.
The article emphasizes the need for awareness and vigilance in addressing such threats, as well as the ongoing efforts to find reliable mitigations in the face of evolving cybersecurity risks posed by AI technologies.
The video delves into the importance of evaluating vulnerabilities in Large Language Models (LLMs) for security and ethical reasons. It explores red-teaming as a methodology to expose these vulnerabilities through case studies and practical examples, distinguishing between structured red team exercises and isolated adversarial attacks.
By providing insights into the types of vulnerabilities uncovered by red teaming and potential mitigation strategies, the talk aims to empower professionals to better assess the security and ethical implications of deploying LLMs in their organizations.
The paper introduces LinkPrompt, a universal adversarial attack algorithm designed to manipulate Prompt-based Fine-tuning Models (PFMs) while maintaining naturalness. Researchers demonstrate that LinkPrompt effectively misleads PFMs into providing incorrect predictions while maintaining the readability and coherence of the generated triggers. Compared to previous methods, LinkPrompt achieves higher attack success rates and demonstrates transferability across different model structures, highlighting the potential vulnerabilities of prompt-based learning paradigms in natural language processing tasks.
The study underscores the importance of developing robust defenses against adversarial attacks on language models and suggests avenues for future research in generating stealthier triggers and applying such methods to broader tasks or larger models.
The video explores the security risks associated with Large Language Model (LLM) architectures and introduces LLM Guard, an open-source tool aimed at enhancing LLM security by screening inputs for malicious intent and outputs for sensitive data.
Demonstrations with models from Hugging Face and OpenAI illustrate LLM Guard’s effectiveness in mitigating risks like prompt injection and unauthorized access to sensitive information, underlining the significance of output monitoring and sanitization for LLM protection. The session underscores the evolving nature of LLM security and emphasizes the crucial role of permissions and monitoring in safeguarding data.
The article explores LLM Red Teaming techniques, emphasizing the importance of understanding AI-specific vulnerabilities and testing methods to improve the security of AI systems. It underscores the need for comprehensive testing against various categories of attacks, highlighting the unique challenges posed by AI applications and the necessity for a diverse skill set in AI Red Teaming.
The integration of Large Language Models (LLMs) presents transformative capabilities in various sectors but also significant security risks, with attackers exploiting vulnerabilities despite ongoing enhancements. Existing studies lack a concise method for assessing these risks, necessitating a proposed risk assessment process utilizing tools like OWASP’s methodology.
By proposing a comprehensive risk assessment process, including scenario analysis and impact assessment, the authors aim to provide security practitioners and decision-makers with actionable insights to effectively mitigate LLM-related risks and enhance overall system security.
Dropbox’s security research team discovered a vulnerability in OpenAI’s ChatGPT models that could be exploited via repeated character sequences in user-controlled prompts, leading to hallucinatory responses. Despite OpenAI’s initial mitigation efforts, Dropbox demonstrated that the models were still vulnerable to repeated token attacks, prompting OpenAI to implement further remediations.
The findings highlight the importance of addressing security vulnerabilities in LLMs, as they can have implications beyond specific models, potentially impacting various commercial and government workflows.
PyRIT (Python Risk Identification Tool) is an open-source framework developed by Microsoft that automates the identification of risks in generative AI systems, enhancing the efficiency of security professionals and machine learning engineers. It enables rapid iteration and testing of prompts and configurations to improve the defenses against prompt injection attacks and other potential security threats in generative AI systems, thereby safeguarding organizations from manipulation and harm caused by bad actors.
The OWASP Top 10 list of security vulnerabilities for LLM applications outlines critical threats like prompt injection, insecure output handling, and training data poisoning, emphasizing the importance of addressing these risks in the adoption of LLMs like OpenAI’s GPT-4 for various business processes. By understanding and mitigating these vulnerabilities, organizations can ensure the security and integrity of their AI systems, safeguarding against potential cyberattacks and data breaches.
The Databricks AI Security Framework (DASF) whitepaper introduces a comprehensive approach to securing AI systems, aiming to improve collaboration among various teams within organizations. By demystifying AI and ML concepts, cataloging security risks, and providing actionable recommendations, DASF assists organizations in mitigating potential security threats associated with the deployment of AI technologies, thus fostering trust and ensuring the responsible adoption of AI.
The key idea of this article is to provide a primer on LLM security, emphasizing the importance of considering security challenges associated with the widespread adoption of LLMs in various applications. It highlights the need for understanding LLMs’ vulnerabilities, the evolving threat landscape, and the implications for security professionals, developers, and society as a whole. Yes, it matters because it addresses the critical need for awareness and proactive measures to secure LLMs and mitigate potential risks in an increasingly AI-driven world.
With the impending activation of the EU AI Act, companies are under pressure to ensure compliance, leading to the development of governance tools to facilitate workflow governance, compliance, and ethical AI practices. This matters because it highlights the growing importance of responsible AI practices and compliance with regulations, driving innovation in the development of tools to address governance challenges and ensure ethical guidelines are met.
Not one, not 10, but 55! LLM Hacking games in one post! On the OpenAI Community.
This job is for an Engineering Manager, Security at Cohere, a company at the forefront of machine learning technology. The role involves building and leading a team of security engineers to develop automated security controls, drive industry collaboration on LLM-specific security research, and manage security operations in cloud-native environments, with a focus on fostering diversity and inclusion.
This job is for a Technical Program Manager in AI Safety at Google DeepMind, involving scoping, planning, and delivering technical programs to advance generative AI goals responsibly. The role requires partnering with internal and external stakeholders, managing risks, and ensuring safety considerations are integrated into AI systems’ design, development, and deployment, with a focus on advancing AI safety and ethics.
The research introduces a program of evaluations to assess the dangerous capabilities of AI systems, covering areas like persuasion, deception, cyber-security, and self-reasoning. While no strong evidence of dangerous capabilities was found in the evaluated models, early warning signs were flagged, highlighting the importance of rigorous evaluation methods to prepare for future AI advancements.
The results are important as they contribute to the development of a systematic approach for evaluating the potential risks posed by AI systems. By identifying early warning signs and suggesting areas for further research, the study helps advance the understanding of AI capabilities and informs strategies for mitigating potential risks associated with AI advancements.
The research introduces SafeDecoding, a safety-aware decoding strategy aimed at protecting LLMs from jailbreak attacks, which can lead to the generation of damaging or biased content. By addressing the limitations of existing defenses and demonstrating superior performance against jailbreak attempts on various LLMs, SafeDecoding offers a promising solution to mitigate the risks associated with hostile inputs, ensuring the continued usefulness of LLMs in benign user interactions.
Researchers have devised ArtPrompt, an ASCII art-based method to jailbreak AI chatbots like ChatGPT, Gemini, Claude, and Llama2, allowing them to respond to malicious queries they’re intended to reject. The research demonstrates the vulnerability of large language models to ArtPrompt-induced attacks, highlighting the need for enhanced safety measures to protect against such exploits.
Written by: admin
Secure AI Weekly admin
X’s Grok AI is great – if you want to know how to hot wire a car, make drugs, or worse The Register, April 2, 2024 The innovative generative AI ...
Adversa AI, Trustworthy AI Research & Advisory