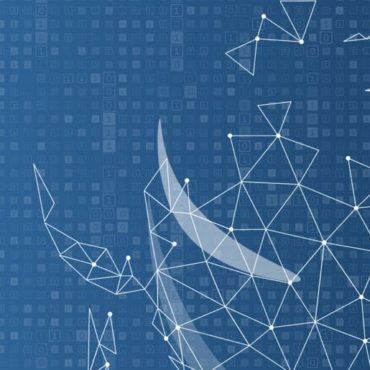
Towards trusted AI Week 25 – New ways to address AI bias
Biased AI can cause significant damage to reputation and business AI Weekly: NIST proposes ways to identify and address AI bias Venture Beat, June 25, 2021 It’s no secret that ...
Adversarial ML admin todayJune 30, 2021 93
Our team makes for you a weekly selection of the best research in the field of artificial intelligence security
In face morphing attacks, a morphed reference image of someone’s face can pass a successful verification as two or more persons. Such attacks can pose a great threat to facial recognition systems. Baaria Chaudhary, Poorya Aghdaie, and Sobhan Soleymani in collaboration with other researchers pay attention to this issue with the help of a face morphing attack detection algorithm and an undecimated 2D Discrete Wavelet Transform (DWT) in their recent study.
The researchers highlight that the artifacts that appear in the face morphing process may not be that noticeable in the image domain, but can be quite easily traced in the spatial frequency domain. At the same time, the disparity between the morphed image and a real one can be highlighted with the help of a discriminative wavelet sub-band.
As part of the research, the authors use Kullback-Liebler Divergence (KLD) for the differences’ exploitation and isolation of the most discriminative sub-bands and make measurements of discrimination of a sub-band is by its KLD value; the 22 sub-bands with the highest KLD values are chosen for network training. After that, a deep Siamese neural network is trained with the help of the 22 selected sub-bands for differential face morphing attack detection and the efficacy of discriminative wavelet sub-bands for morph attack detection gets estimated.
While much attention has been paid to adversarial attacks, most physical attacks are based on the principle that a patch is located on some physical accessory placed on the face. Researchers Kyu-Lim Kim, Jeong-Soo Kim, Seung-Ri Song, Jun-Ho Choi, Chul-Min Joo, Jong-Seok Lee presented for the first time an optical adversarial attack that physically introduces changes into the light field information. In other words, instead of using some accessory with a patch printed, the researchers performed an attack by direct a special light beam towards the camera. As a result, the altered information archives the image sensor tricking the classification model into misclassification.
As part of the attack, the phase of the light in the Fourier domain is modulated with the help of a spatial light modulator located in the photographic system. The presented experiments are based on both simulation and a real hardware optical system. The authors also highlight that the proposed method fundamentally differs from the widespread optical-domain distortions including spherical aberration, defocus, and astigmatism when it comes to both perturbation patterns and classification results.
However, while this is certainly an interesting approach, the results are not perfect enough to speak of a big threat at the moment. However, this direction requires more detailed research and more accurate attacks.
When talking about adversarial attacks, most often they consider either evasion attacks on testing data or poisoning attacks on training data. In recent work, Liam Fowl, Micah Goldblum, Ping-yeh Chiang, Jonas Geiping, Wojtek Czaja and other researchers demonstrate that adversarial examples meant for attacking pre-trained models show more effectiveness in cases with data poisoning than recent methods created exclusively for poisoning do.
According to the researchers, when the original label of their natural base image is assigned to adversarial examples, they cannot be applied to the training of a classifier for natural images. In case the adversarial class label is assigned to adversarial examples, they are useful for training. Hence adversarial examples have important semantic content with the “wrong” labels from the network point of view.
The proposed adversarial poisoning method is said to be significantly more effective than the available poisoning methods for secure dataset release. Also, the researchers propose a poisoned version of ImageNet, ImageNet-P, to emphasize the importance of further research on the issue.
In addition, the use of adversarial attacks for poisoning scenarios was tested a long time ago in the framework of the Adversa AI Red Team research. This is also the main feature of Adversarial Octopus, a universal method of attacks on face recognition systems.
Written by: admin
Secure AI Weekly admin
Biased AI can cause significant damage to reputation and business AI Weekly: NIST proposes ways to identify and address AI bias Venture Beat, June 25, 2021 It’s no secret that ...
Adversa AI, Trustworthy AI Research & Advisory