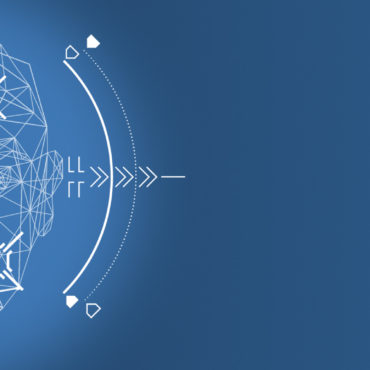
Towards trusted AI Week 23 – The way out of Clearview AI database
One of the main problems of AI is the lack of trust from users Is there any way out of Clearview’s facial recognition database? The Verge, June 9, 2021 Last ...
Adversarial ML admin todayJune 17, 2021 181
Our team makes for you a weekly selection of the best research in the field of artificial intelligence security
Recently adversarial patch attacks have been shown to be effective against the object detection based on deep learning. A specific patch could allow attackers to hide from detectors such as YOLO, which could have serious security implications as it allows attackers to hide from recognition.
In this paper, the researchers Bin Liang, Jiachun Li and Jianjun Huang paid attention to the detection problems, including the object detection and the adversarial patch attacks. As part of the study, the researchers identified a leverageable signature of available patches in terms of the visualization explanation: a signature-based defense method was proven to be effective. Also, the researchers developed an improved patch generation algorithm for estimating the risks in the signature-based way as well as a signature-independent detection method based on the internal content semantics consistency.
As a result it was demonstrated that the signature-independent method can successfully detect existing and improved attacks. According to the authors, both proposed detection methods in combination can offer a comprehensive protection.
It has long been widely known that deep neural networks can be fooled by adversarial attacks when introducing small perturbations and at the moment even adversarial training cannot completely prevent the presence of defense failures.
In this paper, researchers Boxi Wu, Heng Pan, Li Shen, Jindong Gu and others draw attention to the fact that the adversarial attacks can be vulnerable to imperceptible perturbations as well when it comes to adversarially-trained models. Here, perturbing adversarial examples with a small noise can invalidate their misled predictions. As a consequence, researchers are proposing a new method for adversarially trained models of countering attacks with more effective defensive perturbations.
In most cases, fixed datasets are used to validate and test machine learning models, which can sometimes give an incomplete picture of the vulnerability of the models. Sometimes data on vulnerabilities become known only in real attacks, which can lead to real losses for the company.
For this reason, researchers Nataniel Ruiz, Adam Kortylewski, Weichao Qiu and others propose a framework for testing machine learning algorithms with the help of simulators in an adversarial manner. This should help solve the problem of detecting model weaknesses before the model is used in critical systems. It was clearly demonstrated that models often have vulnerabilities that cannot be detected using standard training datasets. As part of this research, the model was studied in a face recognition scenario and it was demonstrated that soft spots of models trained on real data can be found with the help of simulated samples.
Written by: admin
Secure AI Weekly admin
One of the main problems of AI is the lack of trust from users Is there any way out of Clearview’s facial recognition database? The Verge, June 9, 2021 Last ...
Adversa AI, Trustworthy AI Research & Advisory