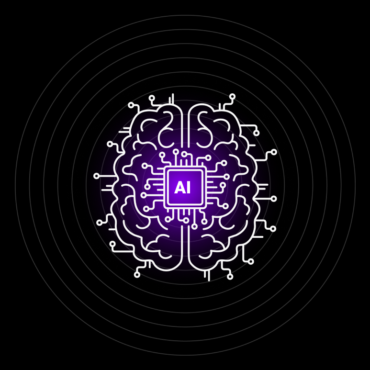
Towards trusted AI Week 46 – how to conceal speech data
The abilities of smart technologies are huge and that is ways it is also both important and difficult to control the risks associated with it. “Smart” stenography for concealing speech ...
Secure AI Weekly admin todayNovember 22, 2020 55
Adversarial attacks with their ability to affect the behaviour of smart systems is something that makes researchers worry and there are reasons why.
TechCentral, November 20, 2020
Much of the recent research has been dedicated to the topic of adversarial examples, and it’s no surprise: adversarial attacks can make an AI-based system perform incorrect actions, and in some cases, for example, when it comes to self-driving vehicles, the results can be fatal. During the year of pandemy, adversarial attacks on ML platforms have even strengthened. According to last year Gartner’s research, over the next two years 30% of all cyberattacks on AI would be adversarial attacks. Sadly, another research by Microsoft demonstrated that the threat of adversarial machine learning is not taken seriously by most industry practitioners.
Still, there is a great need for a certain methodology for detecting and dealing with adversarial risks, and there have already been attempts to reach this goal. For instance, Microsoft, MITRE, and 11 other organisations launched an Adversarial ML Threat Matrix, which is a framework helping specialists classify the most common adversarial tactics applied for disrupting smart systems. The framework highlights four main adversarial tactics used for attacking ML apps and discusses anti-adversarial tactics. Also, the authors provide access to their ‘curated repository of attack’ on GitHub.
TechTalks, November 19, 2020
As ML systems get used more and more widely, currently there’s growing concern about the cybersecurity implications of adversarial examples. AI researchers are busy creating more secure and robust smart systems, adversarial.js, an interactive tool that shows how adversarial attacks work, is among their recent solutions on the way to their goal. Created by Kenny Song, a graduate student at the University of Tokyo, the tool is available on GitHub since last week.The tool is written in Tensorflow.js, the JavaScript version of another deep learning framework, and developer believes that it will help to demystify adversarial attacks and increase awareness about ML security. A demo website hosting adversarial.js has also been launched and here you can make your own adversarial attack by choosing a target deep learning model and a sample image: the image is run through the neural network so that the user can see how it is classified without further adversarial modifications.
“I wanted to make a lightweight demo that can run on a static webpage. Since everything is loaded as JavaScript on the page, it’s really easy for users to inspect the code and tinker around directly in the browser,” Kenny Song explained.
Express Computer, November 20, 2020
The annual State of Cybersecurity Report (SOCR) dedicated to the perspectives of global cybersecurity has been released by Wipro. The report highlights how smart technologies will be applied for defence mechanisms against sophisticated cyberattacks by many organizations. About a half of the companies are working on cognitive detection in their SOCs to stand against unknown attacks.The authors notice that in the last four years there has been a rise in the number of R&D with 49% globally within the cybersecurity filed focused on AI and ML technologies. The report also gives attention to a tendency of shifting towards cyber resilience in the context of remote work during the COVID-19 pandemic.
Written by: admin
Secure AI Weekly admin
The abilities of smart technologies are huge and that is ways it is also both important and difficult to control the risks associated with it. “Smart” stenography for concealing speech ...
Adversa AI, Trustworthy AI Research & Advisory