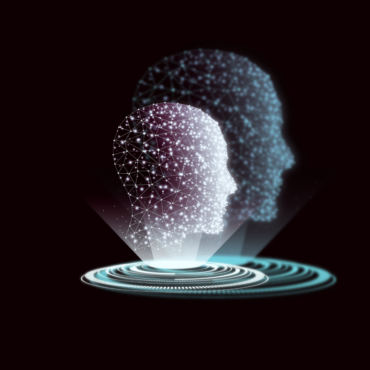
The Greater Good: using AI for public safety
Most uses for AI are not flashy or glamorous: there is rarely a Jarvis ready to prepare an iron suit. Instead, scientists are working on airport security systems, tempering with ...
This is Adversa’s monthly digest of the studies in the field of Machine Learning and Artificial Intelligence. From December 2019 we choose to cover the studies that look at AI applications in our healthcare and public safety systems.
What makes vehicle identification and tracking so complex? Traffic camera footage differs in resolution, scale, and orientation. In general, cars are similar. Yet the same car can look differently depending on perspective and lighting. Suprem et al. suggest turning to teamed classifiers to address these problems. First, a team of functions performs coarse clustering of data based on features such as color or model of a car. Then each cluster is analyzed separately and simultaneously. The initial division into subsets allows for higher accuracy overall. The fact that the classifier is built from smaller models allows it to operate in near real-time. And since the functions are trained independently, the system can be continuously updated with the release of new vehicles.
Universal Adversarial Perturbations for CNN Classifiers in EEG-Based BCIs
Brain-computer interfaces (BCIs) enable people to control wheelchairs and exoskeletons using brain signals recorded by electroencephalograms (EGG). To automate the decoding of those signals scientists suggest using convolutional neural networks (CNNs). However, CNNs are vulnerable to adversarial attacks. Liu, Zhang, and Wu prove that using a highly flexible total loss minimization (TLM) approach it is possible to design universal adversarial perturbations that can be added to any EGG in real-time. This would allow attackers to affect the diagnosis of a disabled person or take control of the user’s wheelchair or exoskeleton, putting them in danger.
Segmentations-Leak: Membership Inference Attacks and Defenses in Semantic Image Segmentation
The availability of large data sets set off the surge in AI development. However, they can be a vulnerability in themselves. If a data set includes private information, models trained using it are susceptible to membership inference attacks, where an adversary can infer whether or not a particular piece of sensitive data was part of the training dataset by looking at the outputs of the model. To protect users He et al. improved upon this class of attacks and then came up with solutions to defer them. Namely, they recommend adding Gaussian noises to data and applying DPSGD to train a model.
CALL TO ACTION
Written by: admin
Adversarial ML admin
Most uses for AI are not flashy or glamorous: there is rarely a Jarvis ready to prepare an iron suit. Instead, scientists are working on airport security systems, tempering with ...
todayApril 13, 2023
Articles admin
Introducing Universal LLM Jailbreak approach. Subscribe for the latest AI Jailbreaks, Attacks and Vulnerabilities If you want more news and valuable insights on a weekly and even daily basis, follow [...]
Adversa AI, Trustworthy AI Research & Advisory